Semi-supervised Acoustic Scene Classification under Domain Shift
Challenge Description:
Acoustic scene classification (ASC) is a crucial research problem in computational audition, and it aims to recognize the unique acoustic characteristics of an environment. One of the challenges of the ASC task is domain shift caused by a distribution gap between training and testing data. Although this task in recent years has achieved substantial progress in device generalization, the challenge of domain shift between different regions, involving characteristics such as time, space, culture, and language, remains insufficiently explored at present. This challenge emphasizes the necessity for ASC models to possess robust performance under domain-shifted conditions. In addition, considering the abundance of unlabeled acoustic scene data in the real world, it is encouraged to study the possible ways to utilize these unlabelled data. We encourage participants to innovate with semi-supervised learning techniques, aiming for more effective use of the abundant real-world data.
Challenge Website:
https://ascchallenge.xshengyun.com/
Organizers
Woon-Seng Gan
Nanyang Technological University, Singapore
ewsgan@ntu.edu.sg
Susanto Rahardja
Northwestern Polytechnical University, China
susantorahardja@ieee.org
Chat-scenario Chinese Lipreading (ChatCLR) Challenge
Challenge Description:
People acquire information through auditory (e.g., voice) and visual (e.g., lip movements) cues to understand spoken content. The audio may be drowned by noise in poor acoustic scenarios, making the content difficult to acquire. Lipreading infers spoken content through lip movements, situated at the intersection of computer vision and natural language processing. Lipreading tasks primarily concentrate on English, emphasizing the need for increased attention in Chinese. The heightened complexity of Chinese lipreading tasks stems from the extensive number of Chinese characters and the complex mapping with the lip movements. The lack of large-scale Chinese lipreading datasets further constrains research progress. Existing Chinese lipreading datasets mainly focus on professional announcers or carefully prepared topics, limiting practical applicability. In contrast, our competition is based on videos recorded in a real-home scenario with 2-6 speakers chatting in a relaxed and unscripted manner.
Task 1: Wake Word Lipreading
Task 2:Target Speaker Lipreading
Challenge Website:
https://mispchallenge.github.io/ICME2024/
Organizers
Low-power Efficient and Accurate Facial-Landmark Detection for Embedded Systems
Challenge Description
In the field of computer vision, facial-landmark detection is crucial for applications like augmented reality and facial recognition. This competition invites participants to develop a lightweight, efficient deep learning model for accurate facial landmark detection across diverse expressions and lighting conditions. The model should be suitable for low-power, real-time performance on embedded systems, especially MediaTek’s Dimensity Series platform. Participants will use the model to identify 51 specific facial landmarks in a test dataset, submitting results as TXT files. Accuracy is assessed based on the mean error, normalized by inter-ocular distance.
Challenge Website:
https://pairlabs.ai/ieee-icme-2024-grand-challenges/
Organizers
Jiun-In Guo
Intelligent Vision System (IVS) Lab, National Yang Ming Chiao Tung University (NYCU),
Chia-Chi Tsai
AI System (AIS) Lab, National Cheng Kung University (NCKU), Taiwan
Multi-Modal Video Reasoning and Analyzing Competition (MMVRAC)
Challenge Description:
Given the enormous amount of multi-modal multi-media information that we encounter in our daily lives (including visuals, sounds, texts, and interactions with their surroundings), we humans process such information with great ease.
For machines to assist us in holistic understanding and analysis of events, or even achieve such sophistication of human intelligence (e.g., Artificial General Intelligence (AGI)), they need to process visual information from real-world videos, alongside complementary audio and textual data, about the events, scenes, objects, actions, and interactions.
Hence, we hope to further advance such developments in multi-modal video reasoning and analyzing for different scenarios and real-world applications through this Grand Challenge using various challenging multi-modal datasets with different types of computer vision tasks (i.e., video grounding, spatiotemporal event grounding, video question answering, sound source localization, person reidentification, attribute recognition, pose estimation, skeleton-based action recognition, spatiotemporal action localization, behavioral graph analysis, animal pose estimation and action recognition).
Challenge Website:
https://sutdcv.github.io/MMVRAC
Organizers
Jun Liu
Singapore University of Technology and Design
Bingquan Shen
DSO National Laboratories and National University of Singapore
Ping Hu
Boston University
Kian Eng Ong
Singapore University of Technology and Design
kianeng_ong@mymail.sutd.edu.sg
Duo Peng
Singapore University of Technology and Design
Haoxuan Qu
Singapore University of Technology and Design
Xun Long Ng
Singapore University of Technology and Design
IEEE ICME 2024 is inviting proposals for its Grand Challenge (GC) program. Please see the following guidelines for this year’s grand challenge submission:
- GC organizers cannot participate as competitors in the GC they are organizing.
- GC organizers should provide a dataset that is open for competition, in particular: i) a training set with ground truth that is available to all participants, and ii) a testing set that is also available to participants, but whose ground truth is hidden. GC organizers are responsible for evaluating all submitted models using the testing set (plus, optionally, a second testing set that is hidden to the participants).
- GC organizers should coordinate reviews of GC papers that describe their submitted models. Submitted GC papers can optionally have a missing results section, which will be completed after GC organizers have completed their evaluations. GC papers have the same camera-ready deadline as regular papers, but a much tighter review cycle. GC paper submission deadline should coincide with the model submission deadline for GCs. Similarly, GC paper acceptance announcements should coincide with the model evaluation results announcement. See below listed dates for details. This gives participants the maximal amount of time to prepare their models for submission to GC organizers for evaluation.
- GC organizers should organize accepted GC papers into their own 2-hour GC session in the ICME’24 program, which should include GC paper presentations (oral or poster), followed by a panel or open discussion. Winners of competitions should be announced during the GC sessions.
- Based on the quality of the accepted GC papers, GC organizers can subsequently submit a proposal for inclusion in a special section of IEEE Transactions on Multimedia via expedited reviews. Further, GC organizers can submit renewal of successfully coordinated GC for the following ICME rendition.
Requirements
Grand Challenge Proposals should follow the requirements below:
- Host organisation
- Coordinator contacts
- Challenge title
- Challenge description (maximum 500 words) and website for further details
- Dataset/APIs/Library URL
- Evaluation criteria (maximum 500 words)
- Deadline submission
- Submission guideline
- Additional information
Submission
Please submit the proposals in PDF format to the relevant Grand Challenge chairs
Important Dates
Grand Challenges proposal submission deadline 15-Dec-23 10-Jan-2024, All submissions are due 11:59PM Pacific time.
Proposal Acceptance Notification 20-Jan-2024.
Grand Challenge Chairs
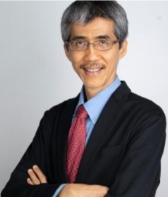
Susanto Rahardja
SIT, Singapore
susantorahardja@ieee.org
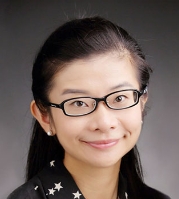
Jiaying Liu
Peking University, China
liujiaying@pku.edu.cn